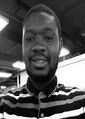
Omisore Olatunji Mumini
Center for Shenzhen Institute of Advanced Technology, China
Title: An online diagnostic model for detecting severity of diabetes
Biography
Biography: Omisore Olatunji Mumini
Abstract
Statement of the Problem: Diabetes is a major health problem that has been found inherent with people of all age groups. As estimated by the International Diabetes Federation in a published report1, 381.8 million of people in the world live with diabetes in 2013, and it has been projected to increase to around to 591.9 million, which is approximately 55% of populationby 2035. Moreover, as one of the top causes of high mortality and morbidity in developing countries, it has impeded the extant purpose of human race. Since medical themes have always advocate earlier detection of diabetes in human as a good medical control, this paper proposes an online procedural model for diagnosis and management of diabetes.
Methodology and Theoretical Orientation: The diagnosis model adopts the fuzzy logic technique to handle imprecise and uncertain information innate with records of diabetes patients. The model was implemented with HTML, Hypertext Preprocessor, JavaScript and XML languages with MySQL taken for backend management. Statistical and sensitivity inferences were drawn from a case study of a dataset from 30 patients, randomly chosen from the patients that were admitted at Obafemi Awolowo University Teaching Hospital Complex, Ile-Ife, Nigeria, between 1994 and 2013.
Findings: Medical data of different groups of people was considered for the experiment and focus was on patients that are overweight and obese. This was because they could be simply assumed pre-diabetic. On running the raw data through the fuzzy logic system, information of the diagnoses was carried out and result gets displayed on screen. For instance, result of Patient P030 confirms that the patient is normal with a severity level of 23.34%. Statistical analysis carried out on the patients’ records shows that nine of the patients were normal, while twenty one were diabetic. 38% of the diabetic patients had mild cases. The data show that others had either severe or very severe cases. Furthermore, 43.3% of the samples were severely diabetic of which 53.8% are male patients and 57.14% of who were above the age of 54 years. Hence, old people who are old are prone to diabetes. Moreover, sensitivity analysis, computed as (TP⁄TP+FN)*100%, the proposed system was 73.3% accurate as the diagnosis recorded from the manual method.
Conclusion and Significance: The need for application of artificial intelligence has been perceived in different areas of medicine. This study presents a fuzzy-based online diagnostic model for detecting the level of diabetes in human. The model demonstrates higher values on statistical and sensitivity analysis. Hence, the model responds aptly to changes in the input values from dataset of the 30 patients considered for this study.